A World Without Work? Don’t Hold Your Breath
Predictions of mass unemployment caused by robots continue to be wildly inaccurateWill we soon be sitting on couches watching reality TV shows while robots work 24/7 doing all of the work humans used to do? The idea that robots will replace most human labor has been around for almost 100 years and has become more popular with each new advance, from sensors and microprocessors to enterprise software, data analytics, and AI.
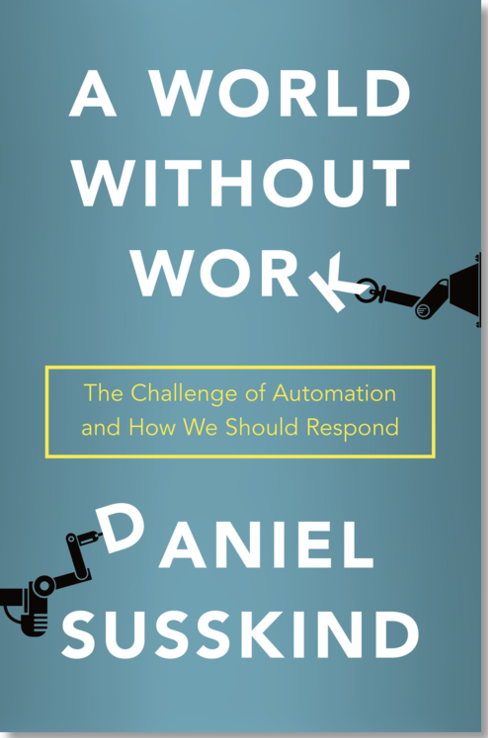
The latest wave of robot hysteria was tweaked by The Singularity is Near in 2005, emboldened by Race Against the Machine in 2012, and sent over the top by The Second Machine Age and The Rise of Robots in 2016, and A World Without Work in 2020, all best sellers. A World Without Work was shortlisted for the Financial Times & McKinsey 2020 Business Book of the Year, in addition to being honored as one of Fortune’s Best Books of the Year, Inc.’s Best Business Books of the Year, and The Times (UK) Best Business Books of the Year. It was a New York Times Book Review Editors’ Choice: “Compelling … Should be required reading for any potential presidential candidate thinking about the economy of the future.”
Articles, books, and documentaries now routinely claim that no job is safe because AI-enabled robots will replace not only farm, factory, retail and transportation workers, but also accountants, journalists, doctors, lawyers, architects, and engineers. We are said to finally be on the threshold of a world in which humans are redundant because all work will be done by robots and computer algorithms.
The reality is that few workers have been replaced by computers. The US unemployment rate was 3.5 percent a year ago, before the pandemic hit, and is now back down to 6.1 percent and falling. Predictions of mass unemployment caused by robots continue to be wildly inaccurate.
There are few self-driving vehicles; Watson Health is being shuttered, Google’s AI efforts are in disarray, and the market for AI services is now predicted to be a fraction of a fraction of what had been confidently predicted five years ago.
Yet prophecies of “A World Without Work” persist. After all, the world’s best chess player is a machine, not a human using a machine, and the same is true in backgammon, Jeopardy, Go, and other games where computers have vanquished humans. Computers evidently do better on their own without human interference.
On the other hand, the Association of National Advertisers chose “AI” as the Marketing Word of the Year in 2017, which certainly makes one wonder how much of the promise of AI is reality and how much is marketing hype.
A simple question can help us understand and anticipate AI’s trajectory: Which applications are succeeding, and which are not? The answer is that applications that augment workers are far more likely to be successful than are those that are intended to replace workers. Watson Health, self-driving vehicles, Open AI’s GPT, and sophisticated chatbots have struggled, while less ambitious projects that augment workers have achieved success by trying to do things that are doable.
The audacious idea that Watson Health could diagnose and treat every illness and disease was breathtakingly arrogant. Playing Jeopardy is very different from being a competent doctor. Even the seductive notion that radiologists will soon be replaced has turned out to be an exaggeration. None have been replaced, few are even being assisted, and researchers continue to disagree about whether AI even has an advantage in interpreting images. One survey found that only one-third of hospitals and imaging centers report using any type of AI “to aid tasks associated with patient care imaging.” The global market for this AI-based imaging software was only $400 million in 2020, a tiny fraction of the $22.8 billion global healthcare software market, despite about $1 billion already spent on R&D. To go from single-case-usage to widespread diffusion will take several years, and the path from assisting to replacing radiologists will take even longerwith most suppliers focused on augmentation not replacement.
Those AI applications in medicine that are successful are generally designed to solve discrete and narrow problems, for example, chatbots that record patient information or alert models for identifying patients who may be at risk for readmission or severe outcomes based on a combination of statistical information and doctor expertise. One expert noted that, “It’s about incremental improvements. It’s not about solving the most complex things in healthcare,” while another cautioned that, “You have to understand where you can insert AI and where it can be helpful without slowing things down in the clinic.” The successes are augmentation, not replacement.
The limitations of AI are revealed clearly in how it handles text. Algorithms can spell-check words, put words in alphabetical order, count the number of times words are used, and other very narrow tasks but have no critical thinking abilities because algorithms literally do not know what words mean. AI algorithms cannot answer this question: “If I were to mix orange juice with milk, would it taste good if I added salt?” Nor can they answer questions like “Who was the American president in 1700?” or “What number comes before 10,000?”
Google Translate’s deep neural network algorithm has improved language translation dramatically, but is still limited by the reality that computers do not truly understand words. Finding matching words in another language, and putting the matches in a grammatically correct order is not the same as understanding context and conveying meaning. This is true not just of translation programs, but of all computer software. Computers can manipulate words and numbers, but do not understand ideas.
Here is a snippet from an article Gary wrote for Fast Company:
Big data, it seems, knows best…. Don’t buy it….Intimidated by the algorithms, humanity could use a little pep talk.
Google Translate’s algorithm translated Gary’s passage into Spanish and back into English:
Big data, it seems, tastes better….I did not buy it….Intimidated by the algorithms, humanity could use a small talk.
The phrase knows best somehow morphed into tastes better. The advice Don’t buy it lost its meaning entirely when it turned into I did not buy it. Finally, pep talk became small talk, two completely different concepts. The passage’s original message was completely lost because Google Translate could not understand what Gary was saying.
This is the fundamental reason why AI programs cannot replace any job that requires critical thinking, wisdom, or common sense. AI programs can compile factoids for writers, but struggle with coherent explanations and reasoning. AI algorithms can be used by lawyers to search for case precedents, but they cannot formulate persuasive arguments for judges and juries. Computer algorithms can be used to grade multiple-choice tests, but cannot formulate challenging questions that require critical thinking. AI algorithms can be used for statistical tests of models predicting sales, expenses, and stock prices, but cannot formulate compelling models to be tested.
…AI programs cannot replace any job that requires critical thinking, wisdom, or common sense.
Computers can give drivers generally accurate directions to their destinations, but self-driving vehicles are only safe to operate along simple, well-defined routes like mining camps, large factories, industrial parks, theme parks, golf clubs, or university campuses—and even then they are either usually monitored remotely or controlled by humans. They are nowhere close to being trusted to travel anywhere in any weather, handling every possible contingency.
A future of augmentation instead of replacement should not be surprising because this is how automation has evolved in farms, factories, and offices. It took 100 years, from 1850 to 1950, for the percent of America’s workers in agriculture to drop from 60% to 20% and another 60 years for those in manufacturing to drop from 30% to 15% (and most of the drop in manufacturing employment came from imports and not automation). Overall, the replacement that occurred came after decades of augmentation.
On farms, the size and speed of plows, seeders, combines, balers, and tractors, and other equipment increased gradually, thereby augmenting the productivity of those operating the machinery. In factories, machine tools were made bigger and faster and gradually connected over time in what was then called computer integrated manufacturing. In offices, computers provided workers with document, spreadsheet, and presentation capabilities that later became enterprise software as computers were connected. Secretarial, data entry, and bookkeeping jobs were lost, but the work of accountants, journalists, engineers, lawyers, and architects were augmented.
The world-without-work enthusiasts disagree. They claim that this time is different, but they have been saying that for decades — most memorably with the New Economy hype that puffed up the dot-com bubble in the 1990s. Yes, computers are faster and cheaper than ever before. Yes, more AI papers are being published than ever before. Yes, an unprecedented number of startups are being funded. Yet, productivity growth is slowing, not accelerating.
Computers are great and getting better, but computer algorithms are still designed to have the very narrow capabilities needed to perform well-defined chores, not the general intelligence needed to deal with unfamiliar situations by assessing what is happening, why it is happening, and what the consequences are of taking action. Humans can apply general knowledge to specific situations and use specific situations to improve their general knowledge. Computers today cannot—which is why we will not see a world without work anytime soon.
In the meantime, let’s use computers to do what computers do best. Computers know more facts than we do, have better memories than we do, can make calculations faster than we can, and do not get tired like we do. This is what make them great assistants — not replacements.